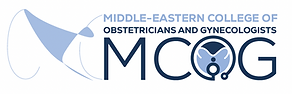
Multicenter
study (MCS) office
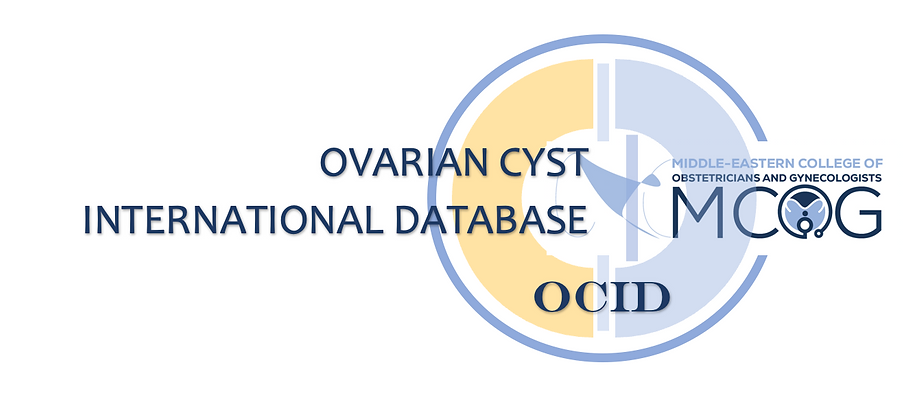
2022
​
​
​
Ovarian cysts are one of the most common gynecologic disorders encountered in
clinical practice. Approximately 20% of women may experience ovarian cysts at least once
in their lifetime (1). However, incidence of significant ovarian cysts is 8% in premenopausal.
In fact, many ovarian cysts are discovered incidentally while pelvic imaging is done for
other indications. Interestingly, prevalence of ovarian cysts amy reach up to 14-18% in
menopausal women, many of which are likely persistent (2). Although most ovarian cysts
are benign, definitive diagnosis cannot be made based on one time sonographic findings.
Simple cysts are typically benign (3). Complex and solid cysts are still likely benign.
However, malignancy is more common in this group of cysts (2). Definitive diagnosis by
histopathology warrants surgical removal of the cyst/ovary. Because the condition is
common and is mostly benign, surgery is not considered unless malignancy is reasonably a
concern or the cyst is symptomatic (4).
Therefore, most ovarian cysts are expectantly managed. Aim of expectant management is to determine cyst changes. Follow-up may extend beyond a year (2). However, recommendations have not been consistent among internationally recognized guidelines, and different cut-offs of cyst size and different frequencies and durations of follow-up were considered (5, 6). Similarly, there are different systems that are adopted by these guidelines to triage women with ovarian cysts based on sonographic and biochemical indicators (5,6).
​
​
​
​
This project aims at creating a prediction model using machine learning algorithms
that can be applied to women with ovarian cysts. The aim of this mode is to determine
probability of cancer and management plan including surgery, long-term or short-term
followup.
​
​
​
​
This is a retrospective cohort study, which will be mediated by multicenter
recruitment. Electronic medical records will be reviewed for “ovarian cyst/mass” code in
both inpatient and outpatient settings between January, 2016 and January 2021.
Retrieved records will be reviewed for eligibility. Patients will be considered for
inclusion if they are postmenarchal, have documented follow-up for at least 1 year
following initial presentation unless surgically managed, and provide authorization to use
their medical records for research purposes. They should have received their care in the
receptive centers. Women will be excluded from the study if they were admitted for an
acute event including cyst torsion, rupture or hemorrhage with no prior documentation of
ovarian cysts. Women with cysts smaller than 3 cm will not be eligible.
A standardized data collection spreadsheet is designed for the purpose of the study
and will be shared with all contributing centers. Data collection will include patient
demographics (e.g., age, parity, body mass index, ethnicity, smoking status), gynecologic
history (e.g., menstrual abnormalities, contraceptive status), medical history (e.g., including
chronic health issues and personal history of cancers), surgical history, family history of
cancers including any diagnosed familial cancer syndromes. Specific information on current
presentation will comprise presenting symptoms, if any, relevant physical signs,
sonographic features (e.g., cyst size, side, consistency, locularity, presence of septa, solid
areas, papillae, intracystic fluid texture, associated pelvic fluid or ascites), features noted in
other imaging modalities if any, tumor markers (CA125, HCG, ALP, LDH,HE-4), management
plan including surgical findings and histopathological diagnosis, follow-up including
follow-up findings and cyst/mass complications during follow-up.
​
Outcomes
The primary outcome of the study is cyst/mass diagnosis as evident by histopathology, cyst resolution or shrinkage over time. Secondary outcomes include incidence of acute events during follow-up, duration of follow-up prior to final diagnosis, and incidence of malignancy in women initially assigned for follow-up and their disease stage. Using machine learning algorithms, a prediction model will be created to calculate individualized risk of cancer in any given ovarian cyst/mass based on patient features, cyst features and biochemical markers, and thereby, further management can be suggested safely.
​
Statistical analysis
Data will be pre-processed prior to final analysis, including data cleaning, imputation
of missing values, dimensionality reduction, and removal of outliers. Data will be utilized as
Xi and Yi where Xi presents input (features) and Yi presents dependent variables
(outcomes). Different classification algorithms will be tested for accuracy to build the final
model including logistic regression, SVM, XGboost and random forest algorithms. Data will
be split at 0.8:0.2 for model training and testing, respectively. Model Validation will be
performed via K-fold cross-validation, and model performance will be tested using
F1-score, precision, recall scores. A learning curve will be used to test for model overfitting
and sample adequacy. Machine learning models will be created using python 3.8®.
Institutional review board (IRB) approval
​
The study meets the criteria of accelerated institutional review board (IRB) review as it conveys minimal risk to research participants.
​
​
​
​
​
-
Mobeen S, Apostol R. Ovarian Cyst. In: StatPearls. StatPearls Publishing, Treasure Island
(FL); 2021. PMID: 32809376. -
Ross EK, Kebria M. Incidental ovarian cysts: When to reassure, when to reassess, when
to refer. Cleveland clinic journal of medicine. 2013 Aug 1;80(8):503-14. -
Johannes Boos, Olga R. Brook, Jieming Fang, Alexander Brook, and Deborah Levine.
Ovarian Cancer: Prevalence in Incidental Simple Adnexal Cysts Initially Identified in CT
Examinations of the Abdomen and Pelvis. Radiology 2018 286:1, 196-204 -
Farghaly SA. Current diagnosis and management of ovarian cysts. Clin Exp Obstet
Gynecol. 2014 Jan 1;41(6):609-12. -
Shazly, S.; Laughlin-Tommaso, S.K. Ovarian Tumors. In Gynecology: A CREOG and Board
Exam Review; Springer International Publishing: Cham, Switzerland, 2020; pp. 489–519. -
Mehasseb MK, Siddiqui NA, Bryden F. The Management of Ovarian Cysts in
Postmenopausal Women. Royal College of Obstetricians and Gynaecologist. RCOG
Green-top Guideline. 2016;34:1-31.
Introduction
Objectives
Materials and methods
References

Dr Angel Yordanov

Dr Erbil Karaman

Dr Ioannis Tsakiridis

Dr Mykhailo Medvediev
Clinic of Oncogynecology
UMHAT "Dr. Georgi Stranski",
Pleven
Bulgaria
Department of Obstetrics and Gynecology
Yuzuncu Yil University, Van - Turkey
Department of Obstetrics and Gynecology
Aristotle University of Thessaloniki - Greece
Dnipropetrovsk Medical Academy of Health Ministry of Ukraine, Dnipropetrovsk, Ukraine
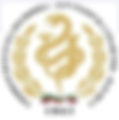
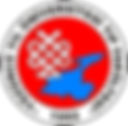
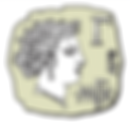
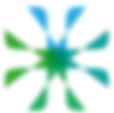
Principle investigator: Sherif Shazly; MBBCh, MSc
sherif.shazly.MOGGE@gmail.com
Study coordinator:
Ahmed Ismail
ahmed.ismail.mogge@gmail.com
Manar Mahran
manar.mahran.mogge@gmail.com
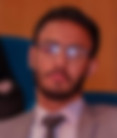
